http://spp.sagepub.com/
Social Psychological and Personality Science
http://spp.sagepub.com/content/early/2013/06/18/1948550613492345
The online version of this article can be found at:
DOI: 10.1177/1948550613492345
Social Psychological and Personality Science published online 18 June 2013
Ryan S. Ritter, Jesse Lee Preston and Ivan Hernandez
Twitter
Happy Tweets: Christians Are Happier, More Socially Connected, and Less Analytical Than Atheists on
Published by:
http://www.sagepublications.com
On behalf of:
Society for Personality and Social Psychology
Association for Research in Personality
European Association of Social Psychology
Society of Experimental and Social Psychology
Additional services and information for Social Psychological and Personality Science can be found at:
Email Alerts: http://spp.sagepub.com/cgi/alerts
Subscriptions: http://spp.sagepub.com/subscriptions
Reprints: http://www.sagepub.com/journalsReprints.nav
Permissions: http://www.sagepub.com/journalsPermissions.nav
What is This?
>> OnlineFirst Version of Record – Jun 18, 2013
Downloaded from spp.sagepub.com at UNIV OF ILLINOIS URBANA on June 20, 2013
Article
Happy Tweets: Christians Are Happier,
More Socially Connected, and Less
Analytical Than Atheists on Twitter
Ryan S. Ritter1, Jesse Lee Preston1, and Ivan Hernandez1
Abstract
We analyze data from nearly 2 million text messages (tweets) across over 16,000 users on Twitter to examine differences
between Christians and atheists in natural language. Analyses reveal that Christians use more positive emotion words and less
negative emotion words than atheists. Moreover, two independent paths predict differences in expressions of happiness:
frequency of words related to an intuitive (vs. analytic) thinking style and frequency of words related to social relationships. These
findings provide the first evidence that the relationship between religion and happiness is partially mediated by thinking style. This
research also provides support for previous laboratory studies and self-report data, suggesting that social connection partially
mediates the relationship between religiosity and happiness. Implications for theory and the future of social science using
computational methods to analyze social media are discussed.
Keywords
Twitter, religion, atheism, happiness, thinking style
Karl Marx (1843/1970) famously asserted that religion is ‘‘the
opium of the people.’’ Though he recognized that religion can
provide comfort in difficult circumstances, for Marx these
benefits were an illusion. The idea that religion hinders true
happiness is echoed by more recent arguments that the world
would be a better place without religion (e.g., Dawkins,
2006; Harris, 2008; Hitchens, 2007). But there is also evidence
for a positive correlation between religion and well-being
(Ferriss, 2002; Hackney & Sanders, 2003; Koenig & Larson,
2001; Poloma & Pendleton, 1990), observed across all four
major world religions (Buddhism, Christianity, Hinduism, and
Islam; Diener, Tay, & Myers, 2011).
In the present research, we use Twitter data to examine two
related research questions: (1) What is the relationship between
religion and happiness? and (2) what particular aspects of
religion contribute to this relationship?We investigate these questions
in a content analysis of Twittermessages (tweets)written by
religious and nonreligious individuals. This approach has several
important advantages. First, unlike traditional studies that assess
happiness through self-report (i.e., directly asking participants
how happy they are or to recall recent positive and negative
emotion; Diener, Suh, Lucas,& Smith, 1999), Twitter data allow
researchers to observe themood of users by the expression of happiness
(or unhappiness) in natural language. Twitter users are not
directed by survey questions or responding in a laboratory setting
that can trigger demand characteristics and distort accurate
responses. Instead, Twitter users are casually conversing on the
Internet with others on topics ranging from the mundane
(e.g., ‘‘I just saw a chicken cross the road’’) to life changing
(e.g., ‘‘I’m getting married!’’). Twitter can therefore provide a
window into users’ state of mind, in real time, as changes and
events are experienced. Furthermore,Twitter.comis currently the
ninth most popular website in the world, yielding millions of
tweets per day from an extremely large and diverse pool of users
(Alexa, 2012). Twitter thus provides a unique opportunity to
study psychological constructs on a large scale that is not possible
through traditional survey and laboratory methods (Lazer et al.,
2009). Finally, content analysis of Twitter allows us to examine
the linguistic markers of numerous different psychological variables
and their interrelationships simultaneously. In the present
research, we investigated two independent mechanisms that may
help explain the association between religion and happiness—
analytical thinking style and social connection—also observable
by differences in language use.
Thinking Style and Social Connection as Mediators
Whether religious people experience more or less happiness is
an important question in itself. But to truly understand how
religion and happiness are related we must also understand why
the two may be related. What features of religion could produce
1 University of Illinois at Urbana–Champaign, Champaign, IL, USA
Corresponding Author:
Ryan S. Ritter, University of Illinois at Urbana–Champaign, 603 East Daniel
Street, Champaign, IL 61820, USA.
Email: ryan.s.ritter@gmail.com
Social Psychological and
Personality Science
00(0) 1-7
ª The Author(s) 2013
Reprints and permission:
sagepub.com/journalsPermissions.nav
DOI: 10.1177/1948550613492345
spps.sagepub.com
Downloaded from spp.sagepub.com at UNIV OF ILLINOIS URBANA on June 20, 2013
differences in happiness? We explored two independent
mechanisms that may mediate the relationship between
religion and happiness. First, believers and nonbelievers may
differ in preference for an intuitive versus analytical thinking
style (Gervais & Norenzayan, 2012; Shenhav, Rand, & Greene,
2011). Whereas intuitive thinking relies on gut feelings to make
decisions, an analytical thinking style emphasizes criticism and
skepticism to draw conclusions (Frederick, 2005). It is easy to see
howdifferences in thinking stylemay be associated with religious
belief. Faith is often characterized by strong emotional conviction
and valued by the very virtue of its uncritical nature. In contrast,
religious disbelief can be characterized by its skeptical approach
to belief. Many scholars suggest that the belief in God is a cognitive
default for humans (Barrett, 2000; Bloom, 2007), and thus
analytical thinking and skepticism may be necessary for one to
reject the dominant belief in God.More important here, however,
these differences in thinking stylemay contribute to differences in
happiness. At its extreme, analytic thinking can foster intense
rumination that can contribute to depression (Andrews & Thomson,
2009).Analytical thinkingmay also diminish the capacity for
optimism and positive self-illusions that typify good mental
health (Taylor & Brown, 1988). If religious people are indeed
happier than nonreligious people, differences in thinking style
may help explain why. But to our knowledge, no previous
research has tested this prediction. Here, we examined whether
nonreligious people exhibit more analytical thinking in their
tweets compared to religious people and whether this could
predict differences in happiness between the two groups.
We were also interested in the role of social relationships as
a mediator between religion and happiness. Several lines of
study have suggested that the quality of social relationships
contribute to overall happiness and well-being (Diener & Seligman,
2002; Lyubomirsky, King, & Diener, 2005; Myers, 2000).
Religion frequently provides a tight-knit moral community in
whom group members can trust and depend on for social
support (Graham & Haidt, 2010). In other words, religious
people benefit by being surrounded by an extended ‘‘family’’
with whom they can share in life’s joys and endure its trials.
Consistent with this idea, religious people report having stronger
social relationships than less religious people, and this
difference in social support predicts happiness (Diener et al.,
2011; Salsman, Brown, Brechting, & Carlson, 2005). Another
goal of the present research was to investigate whether this
effect could be observed in natural language on Twitter.
Method
We report how we determined our sample size, all data
exclusions (if any), all manipulations, and all measures in the
study (Simmons, Nelson, & Simonsohn, 2012).
Procedure
All data were collected using Python v2.7.3, a freely available
and open-source programming language. We gained access to
the Twitter Application Programming Interface using the
Twython package for Python (McGrath, 2012).1
Christian and atheist Twitter users were selected for analysis
by sampling from those who elected to follow the Twitter feeds
of five Christian public figures or five atheist public figures.
The five Christian public figures were Pope Benedict XVI
(@PopeBXVI), Dinesh D’Souza (@DineshDSouza), Joyce
Meyer (@JoyceMeyer), Joel Osteen (@JoelOsteen), and Rick
Warren (@RickWarren). The five atheist public figures were
Richard Dawkins (@RichardDawkins), Sam Harris (@Sam-
HarrisOrg), Christopher Hitchens (@ChrisHitchens), Monica
Salcedo (@Monicks), and Michael Shermer (@MichaelShermer).
The most recent tweet in the sample was from October
1, 2012.
For each of these 10 public figures, we first obtained a list of
their followers and shuffled them into random order. Followers
and their timelines (i.e., recent tweets) were then sampled from
this list at a rate of 150 per hr for a 24-hr period, resulting in
3,600 possible follower timelines per public figure. Only
publicly available follower timelines were accessed and up to
200 of each follower’s most recent tweets were collected. This
process resulted in timelines from a total of 12,849 Christian
followers and 13,367 atheist followers. However, many of
these followers had relatively few tweets in their timeline
and/or did not report English as their language. The final sample
thus included the 7,557 Christian followers (877,537
tweets) and 8,716 atheist followers (1,039,812 tweets) who
self-reported English as their language and had at least 20
tweets in their timeline. Thirteen followers who met all of these
criteria were following both a Christian and an atheist public
figure in our sample and were excluded from the final analysis.
Prior to analysis, each follower timeline was cleaned by
converting all words to lowercase and removing numbers,
hyperlinks, punctuation (except apostrophes), and any mention
of another Twitter user (e.g., @<username>).
The majority of users in our sample either did not self-report
their location (n ¼ 5,252) or reported a time zone in the United
States or Canada (Atlantic ¼ 464; Eastern ¼ 2,377; Central ¼
1,916; Mountain ¼ 472; Arizona ¼ 268; Pacific ¼ 1,238;
Hawaii ¼ 226; and Alaska ¼ 164). The rest of the users
reported locations more sparsely distributed across the world
(e.g., London ¼ 895; Quito ¼ 607; Amsterdam ¼ 263; Beijing
¼ 63; Mumbai ¼ 24; Jerusalem ¼ 14).
Measures
Christian and atheist follower timelines were analyzed using
Linguistic Inquiry and Word Count (LIWC; Pennebaker,
Chung, Ireland, Gonzales, & Booth, 2007), a computerized text
analysis program. Given a piece of text, LIWC counts the
frequency of words or word stems present in a given language
category and outputs the percentage of words that appear in
each category. The LIWC dictionary includes subdictionaries
measuring objective linguistic categories (e.g., pronouns,
articles, and adverbs) as well as a variety of psychological processes
(e.g., affective, cognitive, and perceptual) and personal
2 Social Psychological and Personality Science 00(0)
Downloaded from spp.sagepub.com at UNIV OF ILLINOIS URBANA on June 20, 2013
processes (e.g., work, religion, and leisure). The LIWC dictionary
has been extensively developed and validated (for a
detailed description, see Pennebaker et al., 2007) and has been
successfully applied to the measurement of a wide variety of
constructs (for a review, see Tausczik & Pennebaker, 2010).
For example, LIWC reliably detects the positive and negative
emotion words used when people are asked to write about
positive and negative life events (e.g., Kahn, Tobin, Massey,
& Anderson, 2007) and correlates with human judgments of
affective content (e.g., Pennebaker & Francis, 1996). In a
recent investigation on Twitter, researchers used LIWC to measure
within-person fluctuations in affect and found that people
tend to be happiest early in the mornings and on the weekends
(Golder & Macy, 2011).
Happiness. The presence of positive emotions and the absence
of negative emotions each have an independent influence on
happiness (Diener & Emmons, 1984). Here, we operationalized
happiness as the relative frequency of words in LIWC’s positive
emotion dictionary (e.g., ‘‘love,’’ ‘‘nice’’) to the frequency
of words in the negative emotion dictionary (e.g., ‘‘hurt,’’
‘‘nasty’’). In addition, we examined the independent effects
of positive and negative emotion, respectively.
Social Connection and Thinking Style. Social connection was measured
as the frequency of words in LIWC’s social processes
dictionary (e.g., ‘‘mate,’’ ‘‘friend’’) and analytic thinking was
measured using the dictionary of insight words (e.g., ‘‘think,’’
‘‘consider’’). These dictionaries were developed and validated
using the same procedures as the affective dictionaries
described above (Pennebaker et al., 2007) and have also been
used in previous research (e.g., Pennebaker & Francis, 1996).
Religion. We compared the frequency of words in LIWC’s
religion dictionary (e.g., ‘‘God,’’ ‘‘church’’) to validate our
assumptions about Christian and atheist followers’ own religious
beliefs. The religion dictionary was developed along with
the other LIWC subdictionaries.
To avoid any artificial inflation of association among these
variables, we removed a total of 43 words or word stems that
appeared in more than one of the five LIWC dictionaries of
interest (positive emotion, negative emotion, social processes,
insight, and religion). For example, in the unmodified LIWC
2007 dictionary, the stem ‘‘bless*’’ is included in both the positive
emotion and the religion categories, and the stem ‘‘prais*’’
is included in the social processes, positive emotion, and religion
categories. We therefore excluded these 43 words and
word stems, so that common phrases (e.g., ‘‘praise God,’’ ‘‘God
bless’’) did not artificially bias the results.2
Results
Sample Validation
Table 1 provides descriptive statistics and correlations among
all the variables of interest. Christian and atheist followers did
not differ in the percentage of all words captured by the LIWC
dictionary (grand mean ¼ 74.47, p ¼ .70), suggesting that
differences in linguistic content cannot be accounted for by
simple differences in English proficiency.
As expected, Christian followers tweeted words in LIWC’s
religion dictionary more frequently than atheist followers,
F(1, 16271) ¼ 328.51, p < .001; Cohen’s d ¼ .29, and talking
about religion was associated with less negative affect among
Christian followers (r¼.19, p < .001). Conversely, increased
chatter about religion among atheist followers was associated
with more negative (r ¼ .12, p < .001) and less positive affect
(r¼.09, p < .001). These results suggest that the selection of
Christian/atheist followers was indeed a valid measure of
belief/nonbelief.
Table 1. Descriptive Statistics and Zero-Order Correlations.
LIWC Category M SD Religion Social Processes Happiness Positive Emotion Negative Emotion
Christian followers
Religion 1.12 1.59 —
Social 9.36 3.17 0.18** —
Happiness 3.45 2.68 0.09** 0.22** —
PosEmo 5.53 2.31 0.01 0.34** 0.90** —
NegEmo 2.08 1.16 0.19** 0.16** 0.52** 0.10** —
Insight 1.54 0.78 0.03 0.27** 0.06** 0.05** 0.25**
Atheist followers
Religion 0.73 1.16 —
Social 8.08 2.91 0.14** —
Happiness 2.44 2.25 0.14** 0.11** —
PosEmo 4.77 1.93 0.09** 0.29** 0.86** —
NegEmo 2.33 1.15 0.12** 0.27** 0.51** 0.00 —
Insight 1.78 0.90 0.18** 0.27** 0.08** 0.04* 0.21**
Note. LIWC ¼ Linguistic Inquiry and Word Count; M ¼ mean; SD ¼ standard deviation.
Means are expressed as the percentage of total words within follower time lines. Happiness is operationalized as the difference in negative emotion from positive
emotion.
*p < .01. **p < .001.
Ritter et al. 3
Downloaded from spp.sagepub.com at UNIV OF ILLINOIS URBANA on June 20, 2013
Main Analyses
Because of our large sample, we adopted a significance criterion
of p < .01 for all analyses. We tested a multiple mediator
model using PROCESS with 10,000 bootstrapped samples
(Hayes, 2012), where religious belief (Christian follower ¼ 1,
atheist follower ¼ 0) was used to predict happiness with social
connection and thinking style included as mediators. We also
analyzed the data separately using positive emotion and
negative emotion as outcomes to investigate the independent
components of happiness.
First and foremost, the predicted relationship between
religion and happiness was supported. Relative to the atheist
followers, Christian followers expressed more happiness in
their tweets (total effect ¼ 1.01, standard error [SE] ¼ .04,
t ¼ 26.21, p < .001; Cohen’s d ¼ .41), reflected in the expression
of more positive emotion (total effect ¼ 0.76, SE ¼ .03,
t ¼ 22.88, p < .001; Cohen’s d ¼ .36) and less negative emotion
(total effect¼0.25, SE ¼ .02, t¼13.95, p < .001; Cohen’s
d ¼.22; see Table 1 for means). Second, as seen in Figure 1,
we found evidence that this relationship is partially mediated
by social connection. Christians talked more about social processes
than atheists (b ¼ 1.27, SE ¼ .05, t ¼ 26.73, p < .001;
Cohen’s d ¼ .42), which in turn was associated with more happiness
(b ¼ 0.17, SE ¼ .01, t ¼ 25.51, p < .001; bPositive Emotion
¼ 0.23, SE ¼ .01, t ¼ 42.56, p < .001; bNegative Emotion ¼ 0.06,
SE ¼ .003, t ¼ 21.44, p < .001). On average, 9.36% of words
used by Christian followers were related to social processes,
compared to 8.08% among atheist followers, consistent with
the hypothesis that religion promotes social support and social
connectivity (see Table 1). Indeed, social connection partially
mediated the effect of religious belief on happiness (indirect
effect ¼ 0.21, 99% confidence interval [CI] ¼ [0.17, 0.26];
indirect effectPositive Emotion ¼ 0.29, 99% CI ¼ [0.23, 0.34];
indirect effectNegative Emotion ¼ 0.08, 99% CI ¼ [0.06, 0.10]).
Next, we investigated differences in thinking style. Atheist
followers were more likely than Christian followers to use
‘‘insight’’ words (b ¼ 0.24, SE ¼ .01, t ¼ 17.92, p <
.001; Cohen’s d¼.28), consistent with predictions that atheists
use a more analytical thinking style (see Table 1 for
means). As seen in Figure 1, analytic thinking was then associated
with less happiness (b¼0.36, SE ¼ .02, t¼15.53, p <
.001; bPositive Emotion ¼ 0.11, SE ¼ .02, t ¼ 5.90, p < .001;
bNegative Emotion ¼ 0.25, SE ¼ .01, t ¼ 23.01, p < .001). Use of
insight words also partially mediated the association between
belief and happiness (indirect effect ¼ .09, 99% CI ¼ [.06,
.11]; indirect effectPositive Emotion ¼ 0.03, 99% CI ¼ [0.01,
0.05]; indirect effectNegative Emotion ¼ 0.06, 99% CI ¼
[0.07, 0.05]). Follow-up analyses revealed another meaningful
pattern of thinking style: Christians and atheists differed
in the kinds of insight words used, independent of mean-level
differences. Christian followers were more likely to use insight
words best characterized by certainty and emotion (e.g.,
‘‘know,’’ ‘‘feel’’), whereas atheist followers were more likely
to use insight words characterized by skepticism and analysis
(e.g., ‘‘thought,’’ ‘‘reason;’’ see Figure 2).3 This interpretation
was further supported with follow-up analyses of the LIWC
dictionaries measuring tentativeness (e.g., ‘‘maybe,’’ ‘‘perhaps’’)
and certainty (e.g., ‘‘always,’’ ‘‘never’’). The
percentage of words expressing tentativeness was lower among
Christian tweets (M ¼ 1.70, standard deviation [SD] ¼ .84)
than atheist tweets, M ¼ 2.02, SD ¼ .94; F(1, 16271) ¼
506.72, p < .001; Cohen’s d ¼ .36. On the flip side of this
effect, the percentage of words expressing certainty was higher
among Christian tweets (M ¼ 1.37, SD ¼ .71) than atheist
tweets, M ¼ 1.34, SD ¼ .75; F(1, 16271) ¼ 6.27, p ¼ .01;
Cohen’s d ¼ .04. These findings are consistent with previous
evidence that atheists have a more analytical thinking style,
whereas believers prefer an intuitive thinking style.
Discussion
In a linguistic analysis of nearly 2 million text messages
(tweets) across 16,273 users on Twitter, we found that
Christians express more happiness than atheists in everyday
language. This relation was partially mediated by linguistic
markers of social connection and thinking style. Christians
were more likely to mention social processes that suggest
stronger relationships and support networks. Simultaneously,
atheists were more likely to use ‘‘insight’’ words (e.g., ‘‘think,’’
‘‘reason’’) that in turn predicted decreased happiness, the first
evidence that thinking style partially mediates the relation
between religion and happiness.
Our results reveal important psychological differences
between believers and nonbelievers, and also suggest reasons
why believers may be happier than nonbelievers in general.
However, these findings should not be taken to mean that
religion is a prerequisite for happiness or that atheists are
doomed to be miserable. Religion itself may not provide the
key to happiness. Rather, religion can promote well-being
through other factors. Such insights can be used to improve
happiness in believers and nonbelievers alike. For example,
atheists may improve happiness by creating strong social
0 = Atheist Follower
1 = Chrisan Follower
Social Connecon
(Social)
Analyc Thinking
(Insight)
Happiness
(Posive Emoon)
(Negave Emoon)
1.27**
-0.24**
0.17**
0.72**
(0.44**)
(-0.28**)
(-0.11**)
(0.25**)
(0.23**)
(.06**)
-0.36**
Figure 1. Indirect effects of religious belief on happiness through
social connection and analytic thinking. Values above the paths represent
effects on happiness (positive emotion minus negative emotion);
values in parentheses below the paths represent effects on positive
emotion and negative emotion, respectively. LIWC dictionary names
are in parentheses. LIWC ¼ Linguistic Inquiry and Word Count.
**p < .001.
4 Social Psychological and Personality Science 00(0)
Downloaded from spp.sagepub.com at UNIV OF ILLINOIS URBANA on June 20, 2013
communities and support networks. Currently, atheists are
among the least trusted groups in American society (Gervais,
Shariff, & Norenzayan, 2011) and are bound to experience some
increased level of rumination and unhappiness due to the problem
of social exclusion. However, Atheism and secularism have
increased in recent years (WIN-Gallup International, 2012), and
the divergence in happiness between believers and nonbelievers
may decrease as Atheism becomes more normative. Indeed,
nonreligious people are equally happy as religious people in nonreligious
nations (i.e., where they fit in; Diener et al., 2011), and
increasing the perceived prevalence of atheism can decrease
anti-atheist prejudice (Gervais, 2011). In other words, increases
in happiness among nonbelievers should parallel increases in the
availability of secular social support resources and increased
feelings of being respected in society, both of which facilitate
increased happiness. Future research measuring Twitter activity
in specific regions or nations (e.g., using self-reported location
information along with geotagged information about the precise
latitude and longitude of tweets) is encouraged to examine
questions related to person–culture fit.
It is important to note that there may be other mediators
and variables that account for the relationship between religion
and happiness that are not captured by these particular
analyses. For example, religion may help provide a meaning
system to believers that resolves existential issues and helps
buffer against anxiety (Inzlicht, Tullett, & Good, 2011),
which is consistent with previous evidence that having purpose
or meaning in life also mediates the association between
religion and happiness (Diener et al., 2011). Here, proclivity
for analytic thinking could hurt or help well-being. Atheists
may come to some unpleasant conclusions on existential
issues through analytical thinking, but they may also derive
happiness and meaning from science as an elegant system
of explanation (Preston, 2011; Preston & Epley, 2009). Additionally,
because we measured associations among these variables
simultaneously, we must be very cautious in interpreting
causality. The associations reported may indeed be mutually
reinforcing and could have causal influences opposite the
directions modeled here. For example, having a strong social
support network and meaningful relationships may cause happiness,
but being happy also causes people to have better
social relationships (Lyubomirsky et al., 2005). Future
research could address these limitations of causal inference
by including time as a variable, or by complementing Twitter
analyses with traditional laboratory-based research methods
that afford more experimental control.
The present studies demonstrated powerful effects by
accessing millions of messages available on Twitter. This
Figure 2. Top 30 differences in usage for words within the LIWC insight dictionary. To create this visualization, we first calculated the
percentage usage of each word within the LIWC insight dictionary for both Christian and atheist followers. We next subtracted the atheist
follower percentage from the Christian follower percentage for each word. Finally, we selected the 30 most divergent words for visualization:
15 representing those used relatively more often by Christian followers and 15 representing those used relatively more often by atheist
followers. The sizes of the circles are scaled to represent overall word usage. The color of the circles and their position along the x-axis are
scaled to represent relative word usage among Christian and atheist followers. Values indicate the number of mentions per 100,000 words,
Christian Count–atheist Count. LIWC ¼ Linguistic Inquiry and Word Count
Ritter et al. 5
Downloaded from spp.sagepub.com at UNIV OF ILLINOIS URBANA on June 20, 2013
novel method allows meaningful patterns to emerge in the
specific words people choose to use in tweets, rather than
relying on more traditional self-report methods. Twitter has
considerable advantages as a source of data—its massive scale,
ease of access, high external validity, and fewer demand
characteristics. But of course, it is not without limitations. First,
Twitter users may still engage in some impression management
strategies. Sampling moments are not random and users can
decide exactly what they want to tweet about and when, meaning
people can selectively control the content they want others
to see. This concern is at least partially alleviated by the fact
that Twitter users have no way to know what kind of research
their data may be used for, if at all. There is thus little concern
about the expectations of an experimenter and the impressions
one might make on them.
It is also important to acknowledge that sampling from followers
of major public figures—particularly those on the far
extremes of religious belief and disbelief—may not represent
typical Christians or atheists, and these effects could reflect a
comparison of extremely conservative Christians to militant
atheists. We have also operationalized Christians and atheists
as those who chose to follow public figures well known for
their beliefs. But of course, people can follow these public
figures for reasons wholly unrelated to their religion. Despite
the imperfect nature of this sampling method, the large-scale
nature of Twitter data appears robust. Given that we randomly
sampled from literally millions of possible followers, it is
reasonable to expect a distribution that includes extreme
believers and nonbelievers as well as those with more moderate
or indifferent attitudes toward religion. Most importantly,
we are encouraged by the utility of Twitter data insofar as it
corroborates previous research that has used both laboratorybased
experimental studies (e.g., Shenhav et al., 2011) and
nationally representative samples (Diener et al., 2011). This
convergence suggests that the present findings are not limited
solely to Christian and atheist extremists and that Twitter can
be used to derive novel insights into a variety of phenomenon
of interest to social psychologists.
A final important limitation of the present research—but
one that is not unique to Twitter data—is the inherent limitation
of computerized text analysis. The analyses here relied on
simple word counts, and cannot account for complex features
of language such irony or sarcasm, and are insensitive to context
(e.g., Tweeting about positive things even when unhappy).
People may also negate their use of positive or negative affect
words (e.g., ‘‘not good’’ and ‘‘not bad’’) to convey a valence
opposite to what would be coded by a computer. To address
this possibility, we removed from user’s Twitter time lines all
instances of words in the positive and negative emotion
dictionaries that were preceded by ‘‘no’’ or ‘‘not’’ (see also,
Golder & Macy, 2011). Rerunning the analyses on these data
did not significantly alter the results. Thus, despite some
important limitations of Twitter data, we argue that the benefits
of using computational methods to access large-scale ‘‘realworld’’
data far outweigh the costs, especially when complemented
by more traditional research methods.
Conclusion
Overall, the present research demonstrates a positive relationship
between religion and happiness that can be observed in
subtle differences in language use. This research also sheds
light on some of the underlying reasons for this relationship,
that is, that religious people have stronger social connections
that can promote positive well-being and that atheists engage
in a more analytical thinking style that can diminish
well-being. More broadly, these results reveal the power of
Twitter data as an important research tool. Linguistic markers
of psychological phenomena reliably emerge even in casual
Internet conversations. Twitter data can provide valuable
insight into complex psychological processes and should be
considered a powerful tool for social scientists as people
increasingly live their lives online.
Authors’ Note
The opinions expressed in this publication are those of the authors and
do not necessarily reflect those of the John Templeton Foundation.
Declaration of Conflicting Interests
The author(s) declared no potential conflicts of interest with respect to
the research, authorship, and/or publication of this article.
Funding
The author(s) disclosed receipt of the following financial support for the
research, authorship, and/or publication of this article: This publication
was made possible in part from grant support from the John Templeton
Foundation [grant number 29104], awarded to Jesse Preston.
Notes
1. Example Python code can be obtained from the first author upon
request.
2. Running the analyses using the unmodified Linguistic Inquiry and
Word Count (LIWC) 2007 dictionary yields the same pattern of
results. The biggest difference in using the unmodified dictionary
is that, among the Christian followers, religion and positive
emotion are positively correlated, r ¼ .17, p < .001.
3. An interactive visualization of within-dictionary differences for all
LIWC dictionaries of interest is available at the following website
(requires Java to view): http://labs.psychology.illinois.edu/pramlab/
SPPS_ForceGraph/
References
Alexa (2012). Twitter.com site info. Retrieved December 19, 2012,
from http://www.alexa.com/siteinfo/twitter.com
Andrews, P. W., & Thomson, J. A. (2009). The bright side of being
blue: Depression as an adaptation for analyzing complex problems.
Psychological Review, 116, 620–654.
Barrett, J. L. (2000). Exploring the natural foundations of religion.
Trends in Cognitive Sciences, 4, 29–34.
Bloom, P. (2007). Religion is natural. Developmental Science, 10,
147–151.
Dawkins, R. (2006). The God delusion. Boston, MA: HoughtonMifflin.
6 Social Psychological and Personality Science 00(0)
Downloaded from spp.sagepub.com at UNIV OF ILLINOIS URBANA on June 20, 2013
Diener, E., & Emmons, R. A. (1984). The independence of positive
and negative affect. Journal of Personality and Social Psychology,
47, 1105–1117.
Diener, E., & Seligman, M. E. P. (2002). Very happy people.
Psychological Science, 13, 81–84.
Diener, E., Suh, E. M., Lucas, R. E., & Smith, H. L. (1999). Subjective
well-being: Three decades of progress. Psychological Bulletin,
125, 276–302.
Diener, E., Tay, L., & Myers, D. G. (2011). The religion paradox: If
religion makes people happy, why are so many dropping out?
Journal of Personality and Social Psychology, 101, 1278–1290.
Ferriss, A. L. (2002). Religion and the quality of life. Journal of
Happiness Studies, 3, 199–215.
Frederick, S. (2005). Cognitive reflection and decision making. The
Journal of Economic Perspectives, 19, 25–42.
Gervais, W. M. (2011). Finding the faithless: Perceived atheist
prevalence reduces anti-atheist prejudice. Personality and Social
Psychology Bulletin, 37, 543–556.
Gervais, W. M., & Norenzayan, A. (2012). Analytic thinking
promotes religious disbelief. Science, 336, 493–496.
Gervais, W. M., Shariff, A., & Norenzayan, A. (2011). Do you believe
in atheists? Distrust is central to anti-atheist prejudice. Journal of
Personality and Social Psychology, 101, 1189–1206.
Golder, S. A., & Macy, M. W. (2011). Diurnal and seasonal mood vary
with work, sleep, and day length across diverse cultures. Science,
333, 1878–1881.
Graham, J., & Haidt, J. (2010). Beyond beliefs: Religions bind
individuals into moral communities. Personality and Social
Psychology Review, 14, 140–150.
Hackney, C. H., & Sanders, G. S. (2003). Religiosity and mental
health: A meta-analysis of recent studies. Journal for the Scientific
Study of Religion, 42, 43–55.
Harris, S. (2008). Letter to a Christian nation. New York, NY: Vintage.
Hayes, A. F. (2012). Process: A versatile computational tool for
observed variable mediation, moderation, and conditional process
modeling [White paper]. Retrieved from http://www.afhayes.com/
public/process2012.pdf
Hitchens, C. (2007). God is not great: How religion poisons
everything. Toronto, Canada: McClelland & Stewart.
Inzlicht, M., Tullett, A. M., & Good, M. (2011). The need to believe:
A neuroscience account of religion as a motivated process.
Religion, Brain & Behavior, 1, 192–212.
Kahn, J. H., Tobin, R. M., Massey, A. E., & Anderson, J. A. (2007).
Measuring emotional expression with the Linguistic Inquiry and
Word Count. The American Journal of Psychology, 120,
263–286.
Koenig, H. G., & Larson, D. B. (2001). Religion and mental health:
Evidence for an association. International Review of Psychiatry,
13, 67–78.
Lazer, D., Pentland, A., Adamic, L., Aral, S., Barabasi, A.-L.,
Brewer, D., . . . Alstyne, M. V. (2009). Computational social
science. Science, 323, 721–723.
Lyubomirsky, S., King, L., & Diener, E. (2005). The benefits of
frequent positive affect: Does happiness lead to success? Psychological
Bulletin, 131, 803–855.
Marx, K. (1843/1970). Critique of Hegel’s ‘‘philosophy of right.’’ In
J. O’Malley (Ed. & Trans.). London, UK: Cambridge University
Press (Original work published 1843, p. 131).
McGrath, R. (2012). Twython 2.5.4. Retrieved December 19, 2012,
from http://pypi.python.org/pypi/twython/
Myers, D. G. (2000). The funds, friends, and faith of happy people.
American Psychologist, 55, 56–67.
Pennebaker, J. W., Chung, C. K., Ireland, M., Gonzales, A., & Booth,
R. J. (2007). The development and psychometric properties of
LIWC2007 [Software manual]. Austin, TX: LIWC.net. Retrieved
from www.liwc.net
Pennebaker, J. W., & Francis, M. E. (1996). Cognitive, emotional, and
language processes in disclosure. Cognition & Emotion, 10,
601–626.
Poloma, M. M., & Pendleton, B. F. (1990). Religious domains and
general well-being. Social Indicators Research, 22, 255–276.
Preston, J. L. (2011). Religion is the opiate of the masses (but science
is the methadone). Religion, Brain, and Behavior, 1, 231–233.
Preston, J., & Epley, N. (2009). Science and God: An automatic
opposition between ultimate explanations. Journal of Experimental
Social Psychology, 45, 238–241.
Salsman, J. M., Brown, T. L., Brechting, E. H., & Carlson, C. R.
(2005). The link between religion and spirituality and psychological
adjustment: The mediating role of optimism and social support.
Personality and Social Psychology Bulletin, 31, 522–535.
Shenhav, A., Rand, D. G., & Greene, J. D. (2011). Divine intuition:
Cognitive style influences belief in God. Journal of Experimental
Psychology: General, 141, 423–428.
Simmons, J., Nelson, L., & Simonsohn, U. (2012). A 21 word solution.
Retrieved October 14, 2012, fromhttp://ssrn.com/abstract¼2160588
or http://dx.doi.org/10.2139/ssrn.2160588
Tausczik, Y. R., & Pennebaker, J. W. (2010). The psychological
meaning of words: LIWC and computerized text analysis
methods. Journal of Language and Social Psychology, 29, 24–54.
Taylor, S. E., & Brown, J. D. (1988). Illusion and well-being: A social
psychological perspective on mental health. Psychological
Bulletin, 103, 193–210.
WIN-Gallup International. (2012). Global index of religiosity and
atheism. Retrieved from http://www.wingia.com/web/files/news/
14/file/14.pdf
Author Biographies
Ryan S. Ritter received his BA from the University of Nevada, Reno,
and MA from the University of Illinois at Urbana–Champaign. He is
currently a PhD candidate in social psychology at the University of
Illinois at Urbana–Champaign.
Jesse Lee Preston received her PhD in social psychology from
Harvard University and is now an assistant professor at the University
of Illinois at Urbana–Champaign.
Ivan Hernandez received his BS from the University of Florida and
MA from the University of Illinois at Urbana–Champaign. He is
currently a PhD candidate in social psychology at the University of
Illinois at Urbana–Champaign.
Ritter et al. 7
Downloaded from spp.sagepub.com at UNIV OF ILLINOIS URBANA on June 20, 2013
What particular aspects of religion contribute to this relationship?
You are here: Home / Uncategorized / What particular aspects of religion contribute to this relationship?
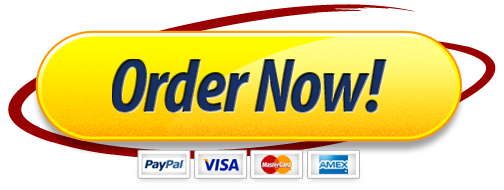
Quick Links
Use Our Writing Service
Our team has experienced writers that follow all the codes used in professionalism when writing academic essays. We focus our services on satisfied clients. Through critical attention to detail, our writers abide by all the instructions given by clients. Additionally, the paper format is done according to the dictates of the client in respect to the set academic style. We are proud of completing outstanding top-quality papers.